Workflow. Confidence. Results.
Greater confidence for better patient care
Not all Research is
created equal
Born from the collaboration of two leading researchers in image processing, ScreenPoint’s very origins required us to be relentlessly focused on pushing frontiers in AI in breast imaging. Clinical research collaborations have been integral to our growth and our credibility.
Workflow
Transpara saves practicing radiologists time - without compromising on clinical quality.
Confidence
Transpara’s unmarked studies have a 99.97% negative predictive value.
Results
Human sensitivity for breast cancer on mammograms can drop from around 80% in the least dense breast tissue to between 50-60% in the densest breasts.
Better Workflow
Whether you are reading DBT studies in a single reader screening environment like the United States, or identifying lower-risk studies for reducing reading burden in a double reading screening environment, Transpara saves practicing radiologists time – without compromising on clinical quality.
Greater confidence
Reading faster has no value without confidence in the results. Transpara’s unmarked studies (70% of a typical screening population) have a 99.97% negative predictive value. These results save ~26% of reading time on DBT Studies (Van Winkel, et al, 2021) or up to 44% of workload in a FFDM Double-reading environment (Lång, et al, 2023)… without compromising – and sometimes improving – cancer detection rate. Save time. Save lives.
Confidence and Results from the most challenging images
One of the barriers to confident mammography reading is breast density. Dense parenchymal tissue is known to obscure indications of cancer on screening mammograms. Human sensitivity for breast cancer on mammograms can drop from around 80% in the least dense breast tissue to between 50-60% in the densest breasts. Transpara analysis is not affected by the visual characteristics of density in the same way.
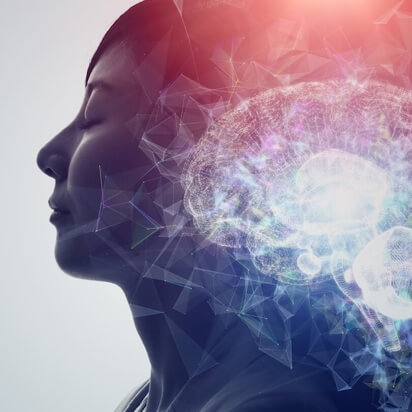
Not all Breast AI is the same
Learn more about how we look at different types of research and how they help us help our users get the best out of Transpara:
Watch the video below
Resources and Bibliography
In 2023, Transpara was the subject of 10 peer-reviewed publications. Clinical users of Transpara are constantly pushing forward with insights into the utility and efficacy of our smart breast AI.
We could give you a lot of reading, but we’re focused on faster workflow, not bigger workload!
If you want all the content, our 2014-2023 compendium bibliography, including educational summaries and links to public access papers can be requested here.
Curious about recent presentations by clinical users and researchers?
You’ll find them in our clinical compendium as well!